WHAT WE HAVE ON THIS PAGE
- Editor’s Choice
- Global Machine Learning Market Share
- Global Market Size of Machine Learning
- Machine Learning in Enterprise Applications
- AI/ML Medical Device Market Growth Forecast
- Model Accuracy and Performance Benchmarks
- Data Volume and Training Trends
- Top Machine Learning Use Cases
- Cloud-Based Machine Learning
- Investment and Funding in ML Startups
- Market Share of Leading Machine Learning Software
- Job Market and Skills Demand in ML
- Top Industries Using Machine Learning
- Ethical and Regulatory Statistics in ML
- Recent Developments in Machine Learning
- Top Machine Learning Adoption Challenges
- Conclusion
- Sources
In a small office in Kansas City, a team of logistics analysts watched as their machine learning dashboard updated in real-time. A year ago, their operation was manually handled by a dozen staff. Today, a few predictive models automatically schedule fleets, detect bottlenecks, and reduce fuel costs, thanks to machine learning. This quiet revolution isn’t unique. In 2025, companies, both massive and modest, are relying on ML not as a futuristic experiment but as a core operational engine.
The surge in adoption, investment, and accuracy is transforming how industries innovate. Whether you’re a data scientist, product manager, or simply a curious mind, these statistics will guide you through what machine learning looks like right now.
Editor’s Choice
- The global machine learning market is expected to reach $192 billion in 2025.
- 72% of US enterprises report that ML is now a standard part of their IT operations, not just an R&D initiative.
- In 2025, 75% of real-time financial transactions will be monitored using ML fraud detection systems.
- Healthcare ML applications in the US saw a 34% year-over-year increase, driven by imaging diagnostics and personalized treatment.
- The average accuracy of leading image recognition models surpassed 98.1% in 2025, narrowing the human-machine performance gap.
- 38% of cloud computing spend in 2025 is attributed to ML training and inference workloads.
- The US ML job market grew by 28% in Q1 2025 alone, outpacing all other tech segments.
- Europe dominates the global machine learning market with a 44.9% share, making it the largest regional contributor.
- North America follows closely with a 44.1% share, showcasing nearly equal influence in the ML landscape.
- The APAC region holds an 11.1% share, reflecting its growing but still emerging role in global ML adoption.
- Combined, Europe and North America account for nearly 89% of the total global machine learning market share.
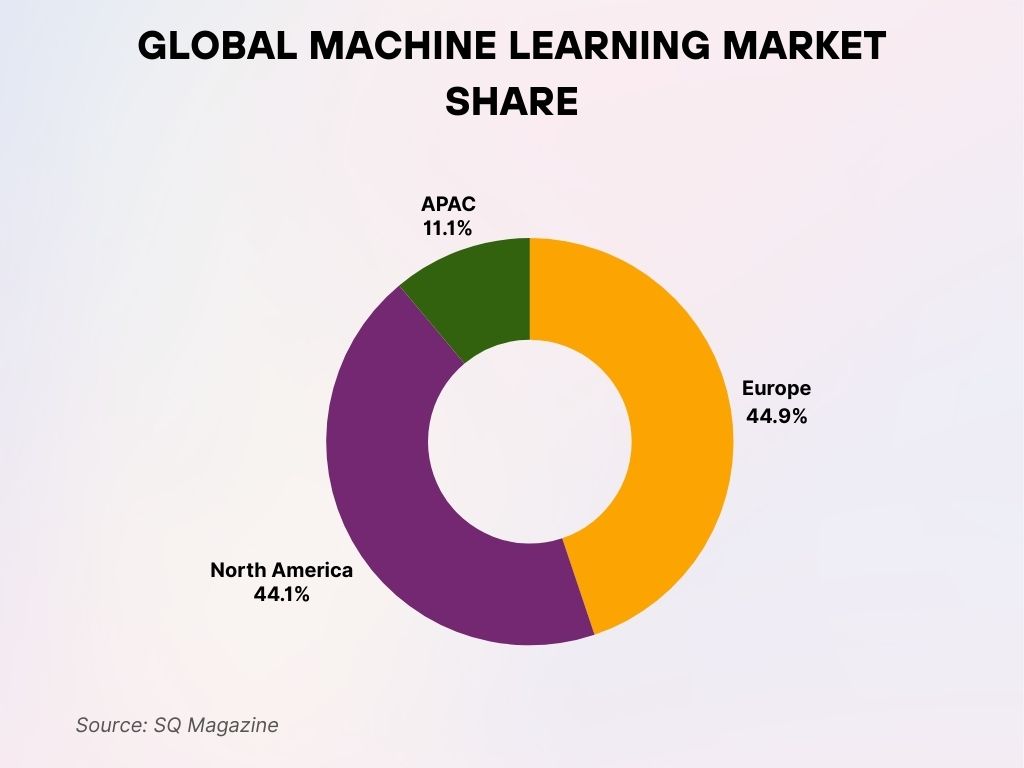
Global Market Size of Machine Learning
- The global machine learning market is projected to reach $192 billion in 2025, a 29.7% increase.
- North America holds the largest market share at 39%, followed by Europe (26%) and Asia-Pacific (24%).
- In 2025, ML software licensing accounts for $72 billion, with platforms-as-a-service (PaaS) contributing $48 billion.
- The enterprise AI market, largely powered by ML, is forecasted to reach $256 billion globally in 2025.
- Government spending on ML infrastructure and research hit $11.4 billion globally in 2025.
- The retail industry’s ML spending globally reached $18.7 billion, driven by customer behavior modeling and logistics automation.
- Cloud-based ML services surpassed $80 billion in 2025 revenue globally, with AWS, Google Cloud, and Azure leading.
- Europe’s ML market grew 31% year-over-year, partly due to aggressive regulatory support and open data initiatives.
- Emerging economies in Latin America and Southeast Asia now make up 9% of the total global ML investment.
- ML adoption is fastest in mid-sized enterprises, which saw a 42% rise in platform onboarding in 2025.
Machine Learning in Enterprise Applications
- As of 2025, 81% of Fortune 500 companies use ML for core enterprise functions like customer service, supply chain, and cybersecurity.
- 55% of all enterprise CRMs now integrate ML-based sentiment and churn analysis tools.
- HR departments across large US enterprises report using ML in 61% of recruitment and talent scoring workflows.
- Document automation powered by ML is deployed in 44% of legal and compliance teams in 2025.
- ML-based inventory optimization systems led to a 23% average reduction in stockouts for large retail companies.
- Enterprise chatbots and virtual assistants using ML handled over 60% of tier-1 customer queries without escalation.
- In finance departments, 38% of forecasting tasks are performed using ML-enhanced predictive models.
- ML is embedded in 72% of ERP systems, often automating invoice processing and vendor performance tracking.
- 29% of B2B SaaS companies now offer ML-based personalization as a service feature.
- ML-powered cybersecurity tools identified and blocked 34% more threats than traditional systems in 2025.
AI/ML Medical Device Market Growth Forecast
- The global AI/ML medical device market is projected to grow from $6.63 billion in 2024 to $21.07 billion by 2029.
- The market is expected to expand at a CAGR of 26.7%, signaling strong and sustained growth.
- In 2025, the market size is estimated to reach $8.17 billion, up from 2024.
- By 2026, growth continues, with projections rising further (specific number not shown but clearly increasing in bar size).
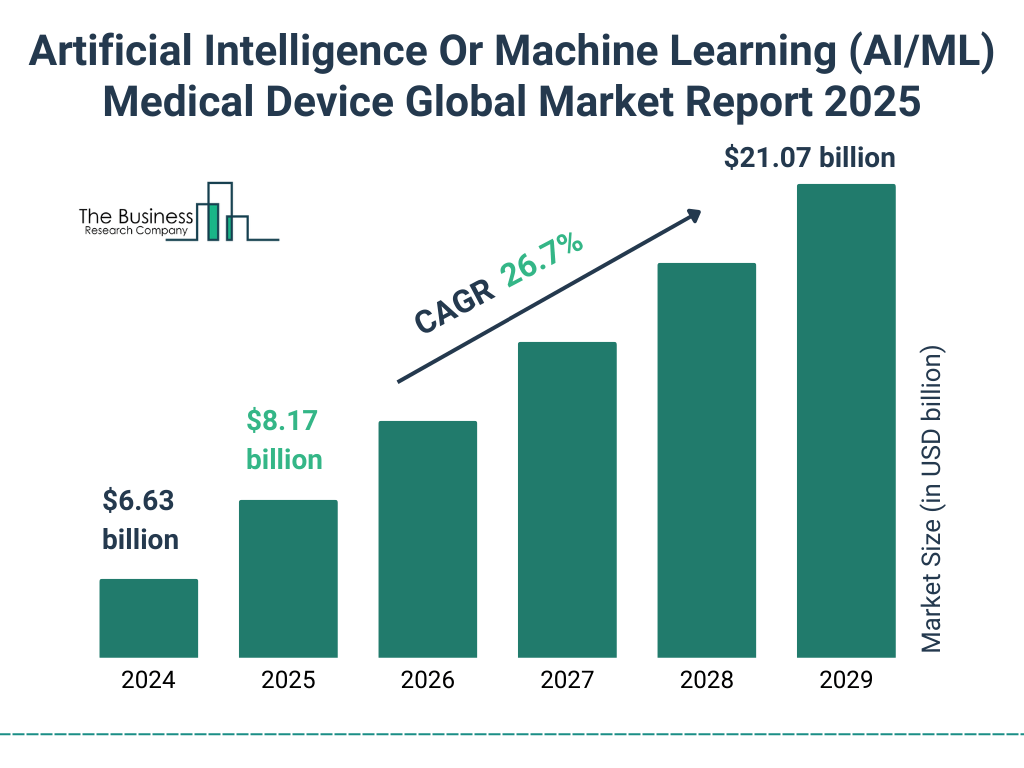
Model Accuracy and Performance Benchmarks
- The average top-5 accuracy for large vision-language models reached 97.3% in 2025.
- Natural language processing (NLP) models surpassed human parity in document summarization tasks with BLEU scores exceeding 45.
- ML models for credit scoring achieved 91% AUC (Area Under Curve) performance, reducing false positives in loan rejections.
- State-of-the-art object detection models now process frames at over 70 FPS on edge devices.
- Speech-to-text models in 2025 have a word error rate (WER) of <4% for general American English, a significant drop from 5.6% last year.
- Federated learning frameworks showed a 13% improvement in convergence speed, even on decentralized datasets.
- AutoML-generated models delivered comparable results to hand-tuned models in 82% of classification tasks.
- In time series forecasting, top ML models reported an average RMSE reduction of 18%.
- Few-shot learning approaches showed 72% accuracy on tasks with under 100 training samples, enabling more nimble ML deployment.
- The top Kaggle-winning solutions in 2025 use model stacking, transformers, and ensemble blending more than any single method.
Data Volume and Training Trends
- In 2025, the average size of training datasets used in enterprise models increased to 2.3 TB, up 40% year-over-year.
- Text datasets are growing fastest, with corporate training corpora exceeding 500 billion tokens on average.
- Synthetic data generation accounts for 23% of training inputs, especially in finance, robotics, and autonomous vehicles.
- Training times for large models were reduced by 31% due to parallelization and distributed GPU clusters.
- Pre-trained model reuse now occurs in 68% of enterprise applications, shortening development cycles significantly.
- Labeled data cost per 1,000 samples dropped to $9.82, due to better data ops and annotation tooling.
- Data augmentation techniques are used in 78% of computer vision projects, particularly in defect detection and surveillance.
- The median ML pipeline retraining frequency in 2025 is every 9 days, reflecting the need for fresher models in fast-changing environments.
- Data versioning tools like DVC and Weights & Biases are used in over 62% of ML engineering teams.
- Bias audits in datasets are mandated in 18 US states, resulting in more equitable training sets and model outcomes.
Top Machine Learning Use Cases
- Reducing costs is the leading ML use case, cited by 38% of respondents.
- Generating customer insights/intelligence ranks second with 37%, emphasizing data-driven decision-making.
- Improving customer experience follows closely at 34%, showcasing ML’s role in personalization.
- 30% of businesses use ML for internal processing automation, enhancing operational efficiency.
- Retaining customers is a priority for 29%, driven by predictive analytics.
- 28% leverage ML for interacting with customers, including chatbots and support tools.
- Recommender systems and fraud detection are used by 27% each.
- 26% focus on reducing customer churn, acquiring new customers, and increasing satisfaction.
- Predicting demand fluctuations is relevant for 25%, aiding inventory and logistics planning.
- Customer loyalty programs are powered by ML for 20% of businesses.
- 19% aim to boost long-term customer engagement using ML strategies.
- Conversion rate optimization is a goal for 17%, aided by predictive modeling.
- Filtering assets/content and building brand awareness are noted by 14% each.
- 15% of respondents mentioned other innovative ML use cases outside the mainstream.
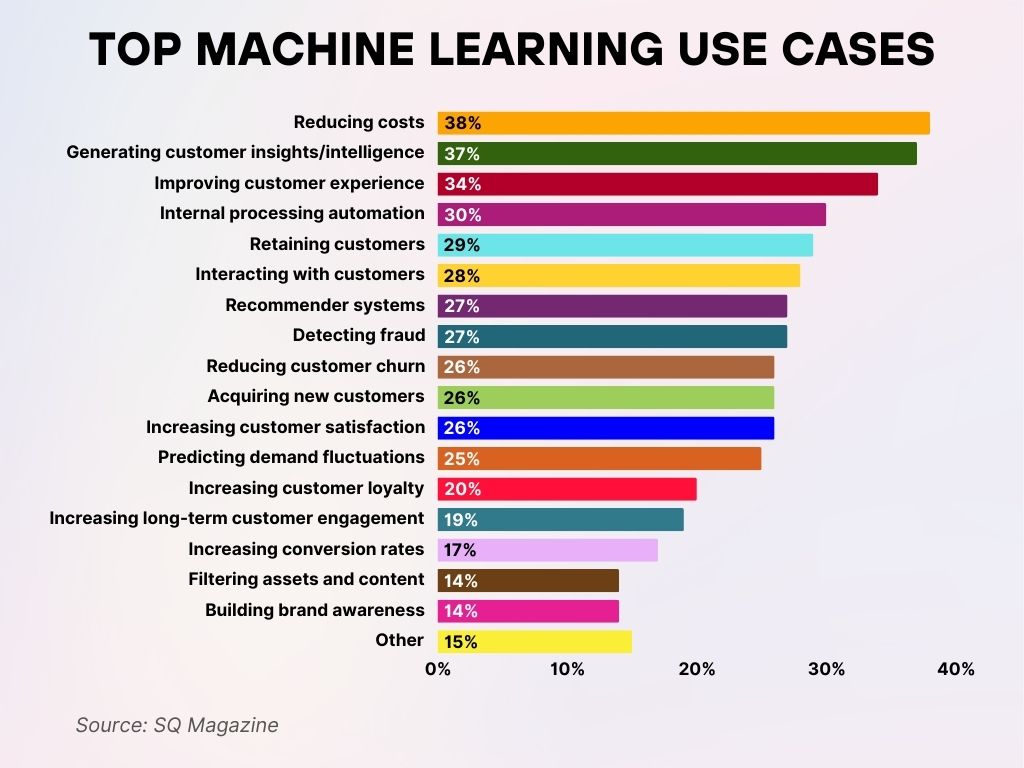
Cloud-Based Machine Learning
- In 2025, 69% of machine learning workloads will run on cloud platforms.
- AWS SageMaker leads with a 32% share of the cloud ML service market, followed by Azure ML (27%) and Google Vertex AI (22%).
- Hybrid ML infrastructures are adopted by 43% of large enterprises, balancing cloud elasticity with on-premise control.
- Serverless ML training use cases grew by 58%, driven by flexibility and better cost scaling for ad-hoc jobs.
- Model inference APIs generated over $6.2 billion in revenue across leading cloud platforms in 2025.
- GPU hour pricing dropped by 15%, making experimentation more accessible for mid-market companies.
- End-to-end ML platforms (e.g., Databricks, DataRobot) are now used by 48% of data science teams for workflow orchestration.
- Auto-scaling ML clusters have reduced idle compute time by 32% on average, improving cloud ROI.
- Cloud providers report that 37% of ML use cases in 2025 will relate to real-time inferencing rather than batch prediction.
- ML model registries and artifact tracking are integrated into 64% of CI/CD pipelines, ensuring reproducibility and compliance.
Investment and Funding in ML Startups
- Global funding for ML-focused startups reached $84.3 billion in 2025.
- US-based ML startups attracted $39 billion, maintaining leadership in global AI investment.
- The average Series B valuation for ML startups rose to $212 million, reflecting investor confidence in scalability.
- ML cybersecurity startups saw a 2025 funding surge of 52%, reaching $8.7 billion, due to rising threats in critical infrastructure.
- AI-driven healthcare startups raised $11.2 billion, with diagnostics, radiology, and genomics as top verticals.
- Venture capital-backed ML startups had a median exit valuation of $540 million in 2025.
- 47% of ML startup founders cited explainability and bias detection as top differentiators for securing funding.
- Corporate venture arms of Big Tech contributed to 19% of all ML investments, indicating strategic alignment with cloud offerings.
- ML startups focused on climate modeling and carbon accounting raised $2.4 billion in 2025.
- Europe-based ML startups accounted for 16% of global funding, with fintech and logistics as leading sectors.
- Newsle dominates the machine learning software market with a massive 88.86% share.
- TensorFlow holds a modest 3.28%, despite its wide recognition in the industry.
- Torch accounts for 2.74%, reflecting a smaller but notable user base.
- Other software collectively represents 5.12%, highlighting the limited diversity in market distribution.
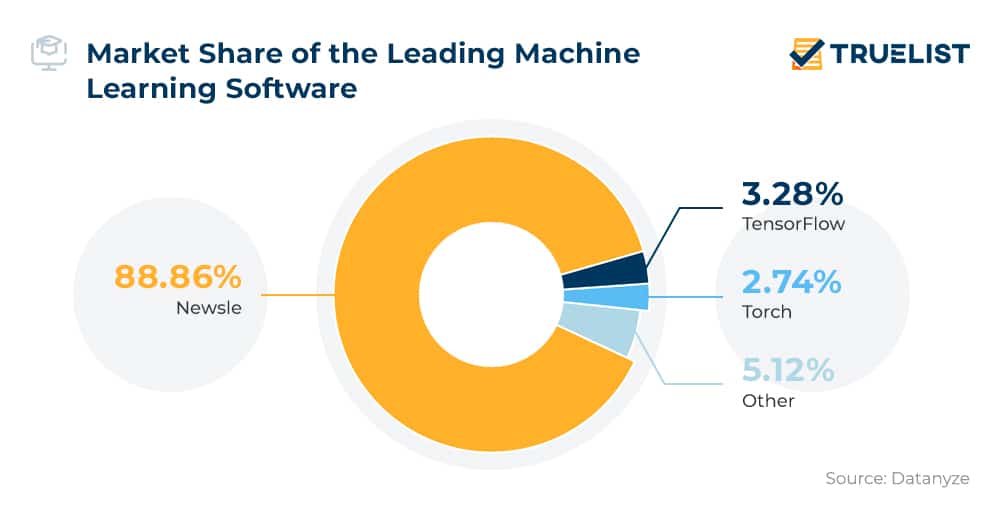
Job Market and Skills Demand in ML
- The ML job market in the US expanded by 28% in Q1 2025, driven by demand for applied data scientists and ML engineers.
- Median base salary for an ML engineer in the US in 2025 is $157,000, with experienced roles exceeding $200,000.
- Python, TensorFlow, and PyTorch remain the top 3 most in-demand ML tools in job postings.
- ML Ops roles grew by 41%, reflecting the demand for model deployment and monitoring expertise.
- 57% of ML job listings now require experience with cloud ML platforms like SageMaker, Vertex AI, or Azure ML.
- Universities in the US reported a 19% increase in ML-related degree enrollments in 2025.
- Freelance ML job platforms saw a 36% increase in contract-based opportunities, especially in small-to-midsize firms.
- Women now make up 26% of ML professionals, marking a 3-point increase.
- Bootcamp graduates represent 17% of new ML hires at mid-sized tech firms in 2025.
- Top hiring sectors include tech, finance, healthcare, and manufacturing, collectively posting over 43,000 ML-related jobs this year.
Top Industries Using Machine Learning
- IT and Telecommunications lead machine learning adoption with 19% usage across the sector.
- Banking, financial services, and insurance closely follow at 18%, highlighting strong reliance on ML for risk, fraud, and automation.
- Automotive and transport use ML in 14% of cases, particularly in autonomous systems and logistics.
- Both the Healthcare and Retail sectors account for 12%, driven by diagnostics, personalization, and inventory systems.
- Manufacturing stands at 10%, leveraging ML for quality control and predictive maintenance.
- Advertising and media use ML in 9% of operations, focusing on targeting and content optimization.
- Other industries make up the remaining 8%, indicating a growing diversity in ML applications.
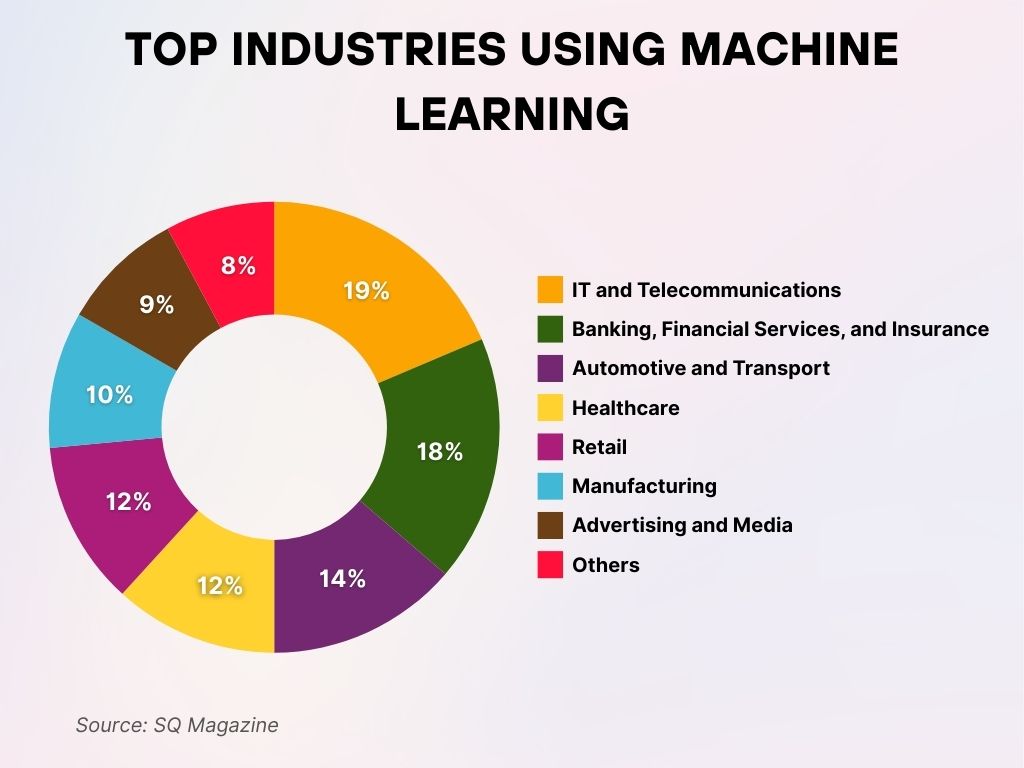
Ethical and Regulatory Statistics in ML
- In 2025, 9 countries have passed AI transparency laws, requiring model explainability in public-facing applications.
- 21 US states have implemented regulatory guidelines for ML model auditing in sensitive sectors like finance and healthcare.
- 47% of US enterprises report conducting regular bias audits on production ML models.
- 78% of AI-focused companies include an ethics compliance section in annual stakeholder reports.
- ML models deployed in public services (e.g., welfare eligibility) are now subject to mandatory fairness checks in 6 US states.
- The EU AI Act, in final implementation by 2025, classifies ML systems by risk level, affecting over 12,000 companies.
- 50% of surveyed data scientists report modifying training data or model parameters based on ethical review feedback.
- ML algorithms used in hiring processes are now required to publish impact assessments in New York, California, and Illinois.
- Open-source ML toolkits for fairness evaluation (e.g., IBM’s AI Fairness 360) are now integrated into 28% of enterprise workflows.
- Public trust in AI sits at 61% in the US, a 4-point improvement, attributed to transparency initiatives.
Recent Developments in Machine Learning
- Transformer-based models are now adapted for multi-modal input (text, image, and audio) in 41% of new research projects.
- TinyML adoption—deploying ML on microcontrollers, grew by 33%, driven by smart home and industrial IoT.
- Quantum machine learning is in proof-of-concept at 8% of large research labs, focusing on molecule simulation and finance.
- Diffusion models used in generative AI are powering 21% of AI-generated media startups in 2025.
- Synthetic data libraries released by government bodies grew to 12 new repositories, enhancing privacy-compliant training options.
- ML model explainability tools now support real-time SHAP value tracking in 22% of enterprise dashboards.
- Reinforcement learning saw a 28% increase in enterprise trials, especially in robotics and logistics.
- Neural architecture search (NAS) is now available in several commercial AutoML platforms, reducing dev cycles by 40%.
- Energy-efficient ML training frameworks are adopted by 37% of organizations with ESG mandates.
- Cross-lingual ML models reached new benchmarks in 2025, offering translation accuracy over 91% across 80+ languages.
Top Machine Learning Adoption Challenges
- Scaling up is the biggest hurdle faced by 43% of organizations adopting ML solutions.
- 41% report issues with versioning and reproducibility in ML models, complicating consistent deployment.
- 34% struggle with organizational alignment and senior management buy-in, affecting implementation momentum.
- 33% cite challenges in supporting cross-programming languages and frameworks, leading to integration issues.
- 28% face duplication of efforts across teams, reducing efficiency and slowing progress.
- 26% fall into the “Other” category, indicating various additional ML adoption obstacles.
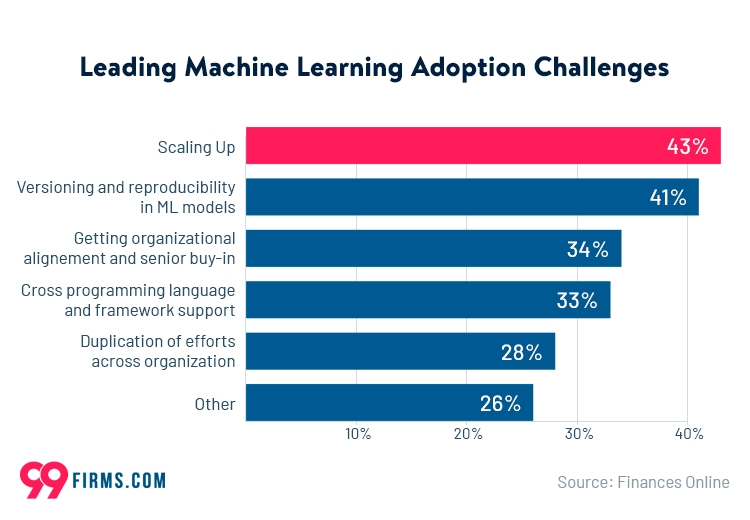
Conclusion
The evolution of machine learning in 2025 is not just a story of numbers, it’s a redefinition of scale, ethics, and everyday integration. From public infrastructure to streaming apps, ML is no longer hidden in the background. It’s in our choices, services, and decisions. And with growing awareness around fairness, explainability, and sustainability, the machine learning landscape is becoming more human-centered than ever before. These statistics illuminate where we stand today and hint at where we’re headed tomorrow.
Sources
- https://www.statista.com/topics/9583/machine-learning/
- https://www.w3schools.com/ai/ai_statistics.asp
- https://www.itransition.com/machine-learning/statistics
- https://www.simplilearn.com/tutorials/machine-learning-tutorial/statistics-for-machine-learning
- https://www.geeksforgeeks.org/statistics-for-machine-learning/
- https://www.datarobot.com/blog/statistics-and-machine-learning-whats-the-difference/
- https://www.datacamp.com/tutorial/unveiling-the-magic-of-statistical-machine-learning
- https://www.frontiersin.org/journals/oncology/articles/10.3389/fonc.2023.1130229/full
ABOUT AUTHOR
